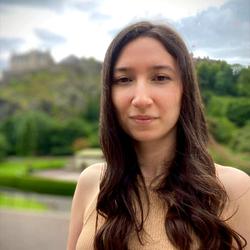
Associating Transcriptomics Data with Inflammatory Markers to Understand Tumour Microenvironment in Hepatocellular Carcinoma
A Talk by Basak Bahcivanci (College of Medical & Dental Sciences, University of Birmingham, Birmingham , UK)
Proudly supported by

About this Talk
Basak Bahcivanci1, Roshan Shafina1, Georgios V. Gkoutos1,2,3,4,5,6, Animesh Acharjee1,2,3,4,
1College of Medical and Dental Sciences, Institute of Cancer and Genomic Sciences, Centre for Computational Biology, University of Birmingham, B15 2TT, UK 2Institute of Translational Medicine, University Hospitals Birmingham NHS, Foundation Trust, B15 2TT, UK 3NIHR Surgical Reconstruction and Microbiology Research Centre, University Hospital Birmingham, Birmingham B15 2WB, UK. 4MRC Health Data Research UK (HDR UK) 5NIHR Experimental Cancer Medicine Centre, B15 2TT, Birmingham, UK 6NIHR Biomedical Research Centre, University Hospital Birmingham, Birmingham, B15 2TT, UK.
Abstract: Introduction: Liver cancer is the fourth leading cause of cancer-related death globally which is estimated to reach more than 1 million deaths a year by 2030. Among liver cancer types, hepatocellular carcinoma (HCC) accounts for approximately 90% of the cases and is known to have a tumour promoting inflammation regardless of its underlying aetiology. However, current promising treatments such as immunotherapy are partially effective for most of the patients due to immunosuppressive nature of tumour microenvironment (TME). Therefore, there is an urgent need to fully understand TME in HCC and discover new immune markers to eliminate resistance to immunotherapy.
Methods: Here, we analyse three microarray data using unsupervised and supervised methods (Fig.1) to discover signature genes. First, univariate, then multivariate feature selection such as Boruta algorithm is applied and followed by an optimization procedure of three combination of dataset pairs with random forest modelling. Resulting optimal gene set is further subjected to network analysis and pathway enrichment analysis to get information about their biological relevance. Moreover, microarray datasets analysed via five deconvolution methods. Consequently, estimation of cell type abundances for each sample in each dataset is achieved.
Results: The AUC values of gene sets obtained in each combination of data pairs range from 0.918 to 0.998 given in the Fig.2. We found 22 genes as an optimum number of genes across three data sets. Among 22 signature genes, LECT2 gene is found as the only common gene as the result of optimization framework. These 22 genes are further investigated with network analysis and pathway enrichment analysis, and we found PLG, KLK1B and C8B genes are to be involved in complement and coagulation cascades pathway (adjusted P-value < 0.001). Cell type abundances between adjacent and tumour samples are compared by using Wilcoxon Rank Sum test which reveales significant changes in Treg cells, M0 and M1 macrophages, and T CD8+ cells (p-values 0.05) among immune deconvolution algorithms. Fig.3 shows CIBERSORT algorithm results of one dataset as an example.
Conclusion: Thereby, we identify two immune related genes, PLG and LECT2, which are recently discovered as the regulators of TME through macrophages and monocytes, respectively. However, experimental validation of the effect of PLG gene on macrophages and LECT2 gene in HCC TME setting is needed. Moreover, immune deconvolution with five algorithms reveals significant infiltration of Treg cells and M0 macrophages, and significant decrease in T CD8+ cells and M1 macrophages in HCC tumour samples.